AI and ML systems are no longer novel technologies in many industries. With increasing adoption, AI/ML development practices and tooling has begun to mature—in fact, an entire ecosystem of open source and proprietary tools has sprung up to support AI/ML developers, dubbed MLOps or Machine Learning Operations software. MLOps practices increase the efficiency, reliability, and general maturity of the ML development process, much like DevOps practices and tooling did the same for software development.
MLOps, however, is narrowly focused on the technical aspects of AI/ML system development and operation. Many of the challenges in the AI space are now seen as fundamentally socio-technical and therefore require integrated, cross-functional solutions. In the face of these challenges, a new field of practice has emerged: AI Governance. AI Governance brings together a diverse range of stakeholders to make decisions about how AI systems should be developed and used repsonsibly to ensure system alignment with business, regulatory, and ethical requirements .
As someone who has worked in both MLOps and AI Governance, I am thrilled to see that these fields are rapidly growing, as they are both crucial to the future success of AI systems. However, they are also incredibly different from one another and are, more often than not, misunderstood concerning how and where they intersect and diverge. At Credo AI, we believe that AI Governance is the missing—and often forgotten—link between MLOps and AI’s success to meet business objectives. In this blog post, we’ll start by defining MLOps and AI Governance, how they differ, and why both are needed for the successful realization of AI/ML projects. Let’s take a closer look at MLOps and AI Governance with respect to scope of work, stakeholder involvement, and development lifecycle.
MLOps vs. AI Governance—what’s the difference?
MLOps: Develop & Deploy ML Models Reliably
Machine Learning Operations, or MLOps, is the set of processes and tools that allow an organization to take an ML model from an idea through development into production. MLOps is focused on solving the technical challenges of ML development and deployment. The primary users and drivers of MLOps within an organization are the data scientists, data engineers, ML engineers, and program managers responsible for building, deploying, and maintaining AI systems within an organization. MLOps sits at the intersection of engineering, data science, and DevOps.
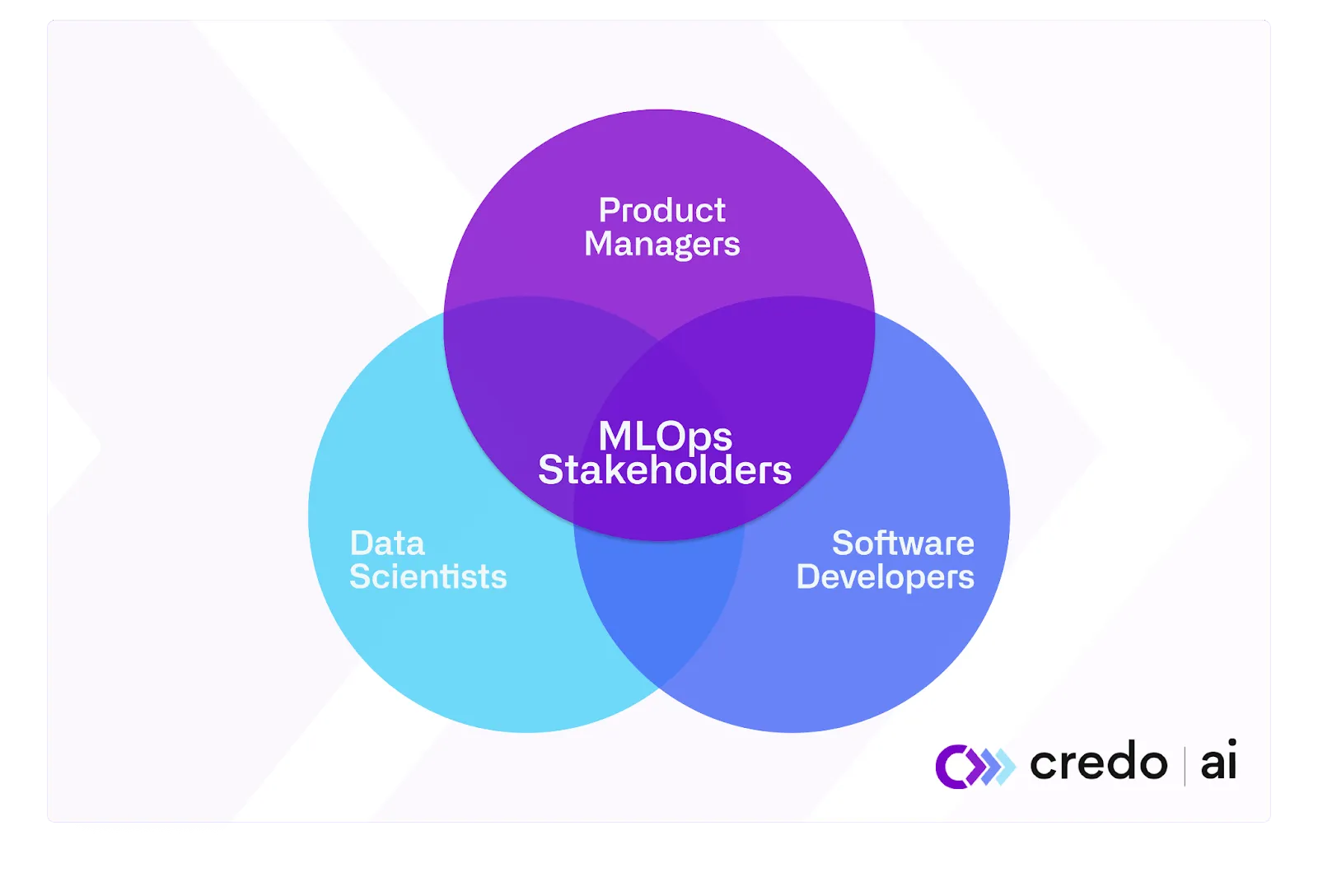
Overall, MLOps is critical to AI/ML development for the same reasons that DevOps is critical to traditional software development; without a set of processes and practices in place to ensure that the ML development lifecycle is repeatable and smooth, AI/ML development cannot scale. The proliferation of MLOps tools and expertise that we have seen recently is a critical step towards AI maturity—MLOps is helping AI to get “out of the lab” and into production at scale.

The standard MLOps lifecycle looks something like this:
Data labeling → data preparation → model training & retraining (experimentation) → model validation → model versioning → model deployment → ongoing monitoring
It is important to note that MLOps activities begin after the decision to build an AI system has been made, and after many decisions have already been made about what the system should do and what the goals or KPIs of the system should be.
MLOps isn’t concerned with the question, “Should we build this?,” but instead is focused on the question, “How can we build this efficiently, reliably, and at scale?”
AI Governance: Measure, Manage, and Mitigate AI Risk
AI Governance is the set of processes, policies, and tools that bring together diverse stakeholders across data science, engineering, compliance, legal, and business teams to ensure that AI systems are built, deployed, used, and managed to maximize benefits and prevent harm. AI Governance allows organizations to align their AI systems with business, legal, and ethical requirements throughout every stage of the ML lifecycle. (For a more thorough definition, please refer to our blog post: What Is AI Governance?)
Data scientists, machine learning engineers, product managers, regulators, lawyers, AI ethicists, business analysts, CTOs, marketers, end users, and impacted communities are all critical participants in AI governance activities and initiatives. The questions that need to be answered during AI governance are inherently interdisciplinary. For example, “How might societal biases show up in this AI system, and what must we do to limit this risk?” is a question that touches on sociology (to understand relevant societal biases based on the use case context), law (to understand the legal or regulatory requirements for fairness of the system or the restrictions on data that can be used to assess and mitigate bias), machine learning (to understand how to measure and mitigate unintended bias), and business (to evaluate any trade-offs associated with mitigation).

At the highest level, AI governance can be broken down into four components—four distinct steps that make up both a linear and iterative process:

- Alignment: identifying and articulating the goals of the AI system.
- Assessment: evaluating the AI system against the aligned goals.
- Translation: turning the outputs of assessment into meaningful insights.
- Mitigation: taking action to prevent failure.
Each step of the governance process requires input based on technical and non-technical expertise from various domains to ensure that all the possible risks and challenges of AI systems are addressed and mitigated. Overall, effective AI governance programs are laser-focused on empowering practitioners with the insights they need to proactively mitigate risk before a catastrophic failure occurs, not after—a vital undertaking reinforced by Dr. Margaret Mitchell, Researcher & Chief Ethics Scientist Hugging Face, at Credo AI’s 2022 Global Responsible AI Summit;"If we don't do due diligence at the start of our products and our AI projects, then we have to correct for issues that emerge afterward.”
AI Governance is concerned with answering the critical questions of “What should we build,” “What are the risks associated with building it,” and “How can we effectively mitigate and manage those risks.”
(To take a deeper look at what happens during each of the four steps of AI Governance, refer to our blog post: How to “do” AI Governance?)
MLOps vs. AI Governance—what’s the overlap?
As you can imagine, both MLOps and AI Governance provide visibility into AI system behavior to allow relevant stakeholders to make better decisions about how those systems should be built, deployed, and maintained. However, while both increase visibility into AI systems, they do so in very different ways.
MLOps gives technical stakeholders critical visibility into AI system behavior—so they can track and manage things like model performance, explainability, robustness, and model bias (performance parity & parity of outcomes) throughout the ML lifecycle. MLOps helps technical stakeholders ensure that a model constantly optimizes its objective function or the technical goal it was designed to achieve.
AI Governance gives a diverse range of stakeholders critical visibility into the risks associated with an AI system—so they can track and manage legal, compliance, business, or ethical issues throughout the ML lifecycle. AI Governance helps a diverse range of stakeholders participate in defining the right objective function for an AI system based on the sociotechnical context in which the system is going to be operating and ensuring that all stakeholders have visibility into whether the system is meeting context-driven requirements to build trust in the system at every stage of the life cycle.
These two different types of visibility are essential to building effective AI systems at scale—and when combined, they allow an organization to deploy AI systems confidently, with the knowledge that any relevant risks—technical and sociotechnical risks have been thoroughly accounted for and effectively mitigated.
How to bring MLOps & AI Governance together.
MLOps provides critical inputs to AI Governance through its infrastructure and tools.
Effective governance requires visibility into AI system behavior. Without MLOps tooling, getting your AI governance team the insights they need to understand and mitigate AI system risk is challenging at best. Having MLOps infrastructure and processes makes the technical evaluation of AI systems against governance-driven requirements much easier and more scalable.
For example, assessing your AI system for bias requires making protected attribute data available to your technical team in the testing or validation environment; without proper data infrastructure and a solid MLOps pipeline, your team will struggle to conduct critical technical assessments required for governance at scale.
Another example: for the “Assessment” results in AI Governance to be trustworthy and auditable, it is critical to maintaining a consistent “system of record” (to track model versions, dataset versions, and the actions taken by the builders of the system). How could you achieve this? With MLOps, and its infrastructure that provides this “system of record,” a critical input for your governance tooling.
As it might be clear now, AI Governance and the four steps of governing AI can only happen at scale with the support of MLOps, and the same can be said for the other way around.
AI Governance provides critical inputs to MLOps.
MLOps tooling makes it easier for technical teams to address technical issues with your models and AI systems, whether it’s adding new data to your training dataset to make your model robust against adversarial attacks or retraining your model using different weights to reduce unintended bias. But without clear guidance on what level of adversarial robustness is required by the system or what kinds of discrimination to test for and mitigate, technical teams won’t know how to make use of MLOps tooling in a way that effectively mitigates relevant AI risks.
For example:
- What constitutes an issue?
- How should this issue be addressed?
- What is the threshold for considering an issue “serious enough” that it requires urgent attention from engineering resources?
These questions are just a few examples of the kinds of questions that cannot be answered by technical stakeholders alone—they need guidance and input from the diverse stakeholders involved in AI governance.
AI Governance provides technical teams with critical inputs to the technical decisions and actions they take during the MLOps lifecycle to ensure that the AI systems they build and deploy meet legal, ethical, and business requirements. With complete clarity and alignment on the principles and metrics that need to be checked, observed, or monitored, technical teams can perform their jobs faster and with less error.
You can ensure that your technical teams have the AI governance insights they need to make the best MLOps decisions by using requirements from regulatory, legal, and ethical guidelines to set thresholds and adjust your objective function during development; connecting your AI Governance platform with the monitoring or alerting system hooked up to your deployment pipeline; and convening diverse stakeholders to make decisions about technical risk mitigation techniques and trade-offs. Governance becomes an enabler of AI innovation and helps build better AI applications.
In Conclusion
If you want to make sure your AI is safe, reliable, compliant, and maximized for benefit, you need both AI Governance and MLOps.
Without MLOps, AI Governance isn’t going to be easily automated or streamlined—and the trustworthiness and auditability of technical insights into model and system behavior will be lacking. Without AI Governance, MLOps are disconnected from the risks that impact your business the most—legal, financial, and brand risk—and your technical teams don’t see the big picture when it comes to detecting and fixing technically-driven AI risk to build better products.
If you’re currently building out your MLOps infrastructure and processes, consider adding a Governance layer to your stack. And if you’re building up your Governance layer but don’t have a strong foundation of MLOps infrastructure, you may want to consider investing in MLOps tooling and skills.
If you need help, reach out to us at demo@credo.ai
DISCLAIMER. The information we provide here is for informational purposes only and is not intended in any way to represent legal advice or a legal opinion that you can rely on. It is your sole responsibility to consult an attorney to resolve any legal issues related to this information.