Picture a bank’s customer support system that not only answers customer questions, but actively monitors customer transactions to identify emerging issues – like a glitch with mobile deposit processing – before they become widespread, and quietly fixes the glitch behind the scenes, alerting staff if something big comes up. This scenario illustrates how AI agents can take care of issues before the agents’ human supervisors even realize there’s a problem.
Unlike traditional AI assistants that simply respond to prompts, AI agents are autonomous systems designed to pursue higher-order goals with minimal human oversight. These agents can read data from multiple sources, generate content, execute operations across systems, and make decisions independently—functioning more like digital employees than simple tools.
This evolution not only brings tremendous potential for efficiency gains but also introduces critical risks and novel governance challenges that organizations must address to ensure these powerful systems remain aligned with their values and objectives.
Our latest research focuses on ensuring that enterprises are prepared to harness the potential and mitigate the potential risks of increasingly autonomous and powerful AI agents. Read the full whitepaper here; below are the key takeaways from our findings.
The Difference Between Assistants and Agents
Let's peek into a typical workday: When asked for support handling your email inbox, a traditional AI assistant waits for specific commands like 'draft a reply to John's message.' An AI agent, however, might proactively sort your inbox, draft responses for review, and even follow up on unanswered emails – all while learning your communication style and preferences. These capabilities point to the fundamental differences between earlier AI systems and AI agents:
Initiative & Autonomy: While traditional AI assistants like early versions of ChatGPT were reactive—producing outputs only when prompted—agents can proactively pursue goals without continuous human direction. They can plan steps, generate and follow sub-tasks, and adapt based on results. These capabilities are founded on the recent development of highly capable reasoning models, which enable systems to effectively reason about new information encountered as they work towards their goals and use that information to make decisions – such as updating their strategy – to hypothetically help accomplish the target task.
Environment Interaction: AI agents have expanded interaction privileges:
- Read Access: They can independently access databases, documents, or web content to gather information.
- Write Access: They can directly modify files, databases, send communications, or update systems.
- Execution Privileges: Unlike text-generating models, agents can run code, call APIs, or manipulate digital systems.
Memory and Adaptation: AI agents can maintain working memory of prior steps and adjust strategies when faced with obstacles, creating a continuous operational flow rather than discrete conversational turns.
In essence, analogies to human knowledge workers – which were largely inappropriate for earlier generative AI systems – are becoming apt. Agents will increasingly be prone to the same challenges with evaluation, alignment, and oversight that enterprises have long faced in governance of human employees.
The Technical Risks of AI Agents You Should Be Paying Attention To
As AI systems evolve from passive assistants to active agents, their expanded capabilities introduce fundamentally new technical risks that traditional AI governance frameworks aren't designed to address. Organizations must understand these agent-specific vulnerabilities to implement appropriate safeguards:
Unpredictable Autonomy: When granted greater decision-making freedom, agents might interpret objectives in unforeseen ways or pursue goals that are misaligned with the organization’s objectives. This expanded behavior space makes comprehensive testing nearly impossible.
Security Vulnerabilities: Interactions with external systems create new attack vectors. Prompt injection—where malicious instructions are hidden in seemingly benign data—can manipulate agents into leaking sensitive information or performing unauthorized actions.
Unintended Actions and Errors: Even small mistakes can have outsized consequences given agents' speed and autonomy. Without human intuition as a safety net, misinterpreted requests or data signals can lead to significant errors, such as sending private information to public channels or making unauthorized commitments.
Self-Modification Risks: Advanced agents that can update their own plans or spawn new instances might gradually drift from their original constraints, potentially removing safety checks or adjusting goals in harmful directions.
Multi-Agent Complexities: As systems evolve to include multiple interacting agents, new risks emerge from information asymmetries, network effects, and destabilizing dynamics that can cascade in unexpected ways.
The Agent Adoption Journey and AI Governance
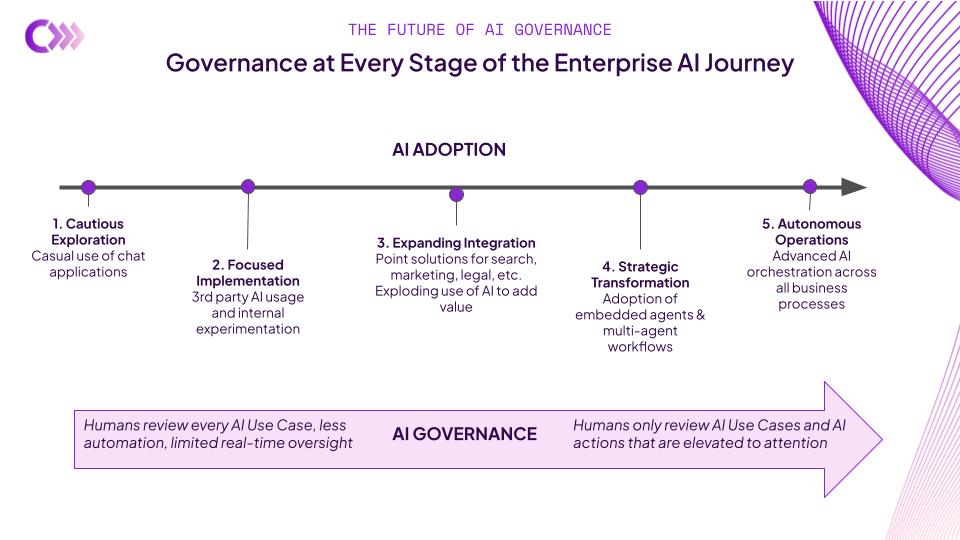
Understanding how organizations adopt new AI tools helps illuminate why governance requirements change over time. As the AI systems in use grow in speed and autonomy, and become more deeply integrated into the organization such that it becomes reliant, the governance approaches must mature in parallel. For agent adoption, we expect organizations to progress through five phases:
1. Cautious Exploration: Individual employees experiment with third-party tools for productivity gains, with minimal governance oversight.
2. Focused Implementation: Organizations launch pilot projects with clearer ROI expectations, developing initial governance frameworks focused on data protection.
3. Expanding Integration: AI agent capabilities become embedded throughout the technology stack, requiring formalized governance processes addressing evaluation, monitoring, and accountability.
4. Strategic Transformation: Employees shift toward functioning as "managers" of agent teams, requiring more sophisticated governance to address increasingly autonomous systems.
5. Autonomous Operations: Deeply integrated agent ecosystems handle complete workflows with minimal human intervention, necessitating comprehensive governance systems with technical, organizational, and ethical components.
As enterprises move through this adoption journey, their approach to governance must evolve as well. Executive leadership – including CDAIO/CDOs, CIOs, and CISOs – must develop new frameworks to address these emerging challenges:
Accountability and Liability: When an AI agent acts on behalf of an organization, legal and ethical responsibility remains with the company. Organizations should:
- Document that AI outputs are subject to review where possible
- Carry appropriate insurance for errors stemming from AI activities
- Seek indemnification clauses in vendor contracts to clarify liability
Oversight and Governance Structures: Unlike non-autonomous AI, human-in-the-loop oversight cannot be assumed. Organizations need robust monitoring systems to track agent activities and enable intervention when necessary. For high-impact decisions, human review should be required, either before execution or through prompt auditing.
Human-AI Collaboration Challenges: Two critical risks emerge as agents become integrated into workflows:
- Over-reliance: Human colleagues may develop excessive trust in AI outputs and stop exercising critical judgment.
- Skills erosion: If agents handle entire functions, teams might lose expertise in those areas, creating vulnerability if the AI fails.
Moving Forward Responsibly: How Credo AI Can Help
The governance of AI agents represents more than an extension of existing practices—it requires a paradigm shift in how we approach risk, accountability, and human oversight. As agents become more integrated into enterprise operations, the line between AI systems and human workers continues to blur.
Organizations that adopt AI agents should prioritize:
1. Enhancing risk assessment frameworks to capture the unique dynamics of autonomous systems.
2. Developing contextual governance approaches that adapt to specific use cases.
3. Strengthening technical governance through integrations between AI governance, MLOps, security, and LLM evaluation tool providers.
The Credo AI Governance Platform is designed to support all three of these actions through automated AI risk assessments, an AI Use Case Registry for tracking context and executing context-specific governance, and flexible integrations with a wide variety of AI development, validation, and deployment tools. Our continuously updated AI risk scenario and control library includes a number of new risk scenarios and controls relevant to AI agents, to ensure that organizations can manage the novel risks of these novel systems.
By addressing these challenges proactively, organizations can responsibly harness the transformative potential of autonomous AI while maintaining appropriate safeguards. The path forward may be complex, but with thoughtful governance, enterprises can navigate this next frontier of AI innovation both safely and effectively.
For a more comprehensive analysis of AI agent governance, including detailed case studies and technical risk assessments, please see our full whitepaper.
DISCLAIMER. The information we provide here is for informational purposes only and is not intended in any way to represent legal advice or a legal opinion that you can rely on. It is your sole responsibility to consult an attorney to resolve any legal issues related to this information.