Most enterprises are struggling to stay afloat with the rapid pace of change and technological innovation that is taking place right now. Generative AI (“GenAI”) is showing up everywhere: in familiar software like Microsoft Office and Slack, specific applications like ChatGPT and Jasper, and as an evolving engineering paradigm and tech stack.
Unlike traditional ML, which has high technical barriers to adoption, genAI is available to anyone.
We at Credo AI have repeatedly heard that companies are missing the visibility and control over GenAI needed for confident adoption. Companies are rightly worried about the risks that this ungoverned proliferation of AI poses to their business.
At the same time, companies know they need to keep pace with these changes to keep up with competitors.
GenAI has the potential to completely transform the way that businesses operate and create value. The ML revolution promised transformation; the generative AI revolution is delivering on that promise. Organizations that aren’t finding ways to augment their employees or business processes with generative AI tools are going to be left behind.
They must find ways to harness the power of this new technology.
There is an incredibly urgent need right now across the enterprise to find ways to govern generative AI. In this blog post, we will summarize the different points of governance for generative AI and provide a market landscape overview of the different tools that are available to enterprises to support the governance of generative AI.
The generative AI stack.
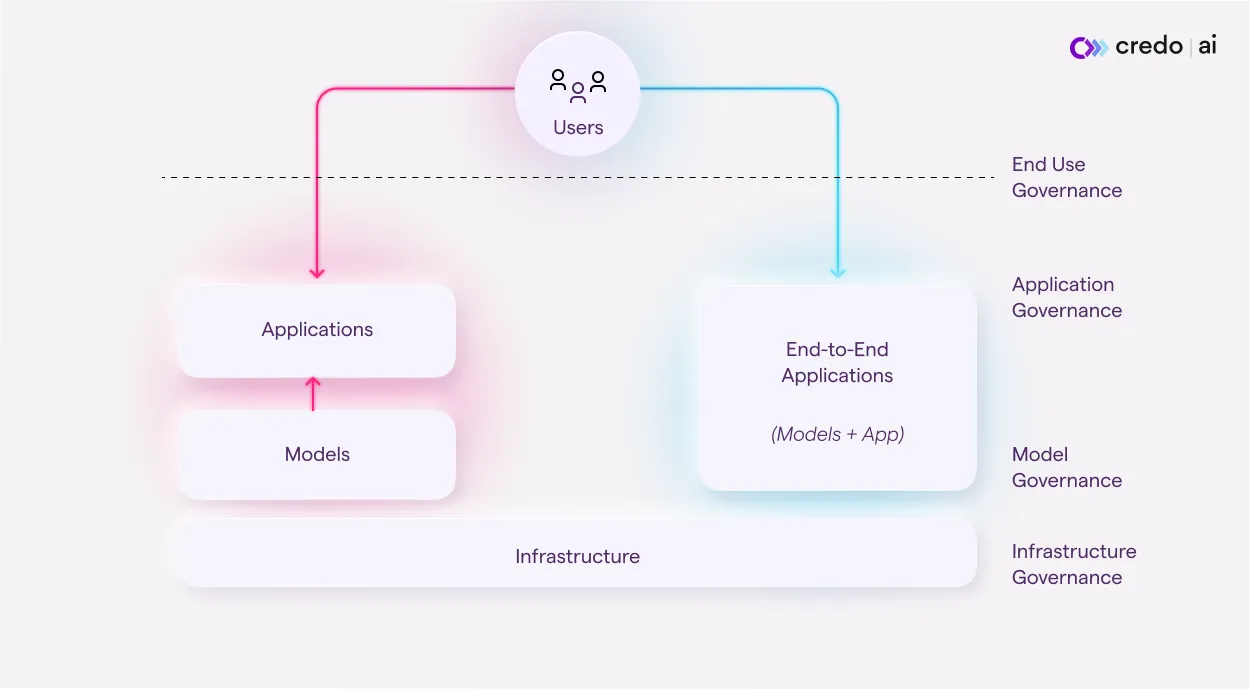
Foundation models—GPT-4, Claude, LLaMa, StableDiffusion, etc.—are at the heart of generative AI tools and systems, but they are only one component of the larger generative AI stack.
Two other critical components are the infrastructure layer—the machines that these models are actually trained and deployed on, which may sit in the cloud or within an organization’s firewall—and the application layer built around or on top of these models, that provides a user interface and tooling for end users.
Up and down this stack, there are opportunities to inject governance and assert control. Let’s look at these governance layers from the bottom up.
Infrastructure governance.
Infrastructure governance—governing generative AI systems by running them on infrastructure that has appropriate security and privacy controls built into it is the only surefire way to mitigate one of the most critical risks of generative AI systems to organizations: leakage of sensitive data or IP.
Deploying generative AI models and applications on secure infrastructure that has appropriate controls for data governance and security is essential to adopting generative AI systems responsibly and safely. Enterprises can quickly reduce the risk of using generative AI tools by ensuring that they’re not using any tools in the public cloud but instead are using tools deployed on infrastructure where they have full control over the data flows.
Model governance.
Model governance, or the policies and processes that control the design, development, and deployment of AI models, is nothing new. Many organizations have been doing some form of model risk management for years. In the era of generative AI, however, enterprise model governance looks very different because most enterprises aren’t building their own foundation models.
Instead, they are relying on foundation model providers like OpenAI and Anthropic.
These third-party providers are increasingly investing in tools and processes to manage and mitigate privacy, safety, and security risks at the model level—these investments include foundation model evaluations to better quantify model behavior and “alignment” approaches like reinforcement learning through human feedback and constitutional AI, which reduce the likelihood of common failure modes and improve model steerability.
These safeguards, however, are not tailored to any particular use of foundation models, nor are they grounded in a specific industry or organization’s risk tolerance and compliance needs. Enterprises that have a low-risk tolerance or specific concerns related to a particular application of foundation models are finding that the model governance of third-party providers is not sufficient for their needs.
For these organizations, there are two options: explore the use of open-source models to enhance their control over the model or implement stronger layers of governance on top of and underneath the model in the other layers of the GenAI stack.
Application layer governance.
The application layer provides the user interface for generative AI APIs, and so there is a tremendous opportunity to insert governance controls into this layer to prevent a foundation model from being used in dangerous or non-compliant ways.
By their nature, genAI systems are more flexible and difficult to predict than traditional software engineering, which presents new challenges for application builders. For example, GenAI applications are vulnerable to prompt injections and misuse by malicious users.
It is also easy for genAI applications to return outputs that are harmful or in violation of governance policies and requirements. These issues can generally be dealt with by input/output (I/O) governance, where safeguards (e.g., automatic moderation) are added around foundation model API calls to reduce risks.
We’ve seen this approach used in the very application that made generative AI a household name: ChatGPT.
While ChatGPT sits upon a foundation model, it is actually an application with multiple components. Some of these components are designed to provide a layer of governance on top of the GPT model to reduce the risks associated with its use.
For example, ChatGPT “plugins” address gaps in the underlying model’s capabilities to reduce the risk of incorrect outputs (e.g., performing arithmetic with a calculator plugin). ChatGPT also implements a set of filters for toxicity and other restricted content to reduce the likelihood that it will be misused by end users.
Adding these kinds of governance controls to the application layer of the generative AI stack is a very effective way to reduce the risk of these systems; however, if an organization isn’t building its own generative AI applications, it still doesn’t have control over this layer.
The last (or, for most enterprises, the first) line of defense: end-user governance.
Without direct control over models or the application layer, what capacity do enterprises have to govern these systems and mitigate against their most egregious risks? For most enterprises, the first line of defense against generative AI risk is end-user governance—governing the ways that end users are allowed to interact with generative AI systems.
Many enterprises responded to the generative AI revolution by implementing the bluntest instrument when it comes to end-user governance: turning off end-user access.
Of course, turning off access to tools like ChatGPT is an effective way to make sure that your employees aren’t exposing your organization to risk via usage; however, it also blocks your organization from realizing the many benefits and obtaining value from these tools.
Examples of end-user governance that allows for safe and responsible exploration of generative AI include adopting a code of conduct that defines how users are and are not allowed to interact with generative AI tools; logging end-user interactions, and monitoring for risky or edge case inputs and outputs; implementing human-in-the-loop review that prevents generative AI outputs from being used without human feedback or input; and enabling users to share effective prompts with one another, so they can become better at successfully using generative AI tools.
Surveying the Market Landscape of Tools for GenAI Governance
Mitigating the risks of generative AI systems requires action across the entire generative AI stack, from the infrastructure to the model to the application layer, as well as interventions at the point of use.
Every day, new tools are popping up that address specific governance concerns at specific points of the stack, from assessing and fine-tuning models to implementing use-case- and industry-specific input/output filters.
We at Credo AI are excited to open-source our internal list of GenAI tools and technologies. This evolving database is your one-stop-shop for a curated list of genAI tools. As you can see, there are many different tools that are all playing in different layers of the stack. Bringing these tools together to insert governance and control across the entire stack is essential to effectively managing and mitigating generative AI risk.
This is not something that one person can solve alone but something that requires collaboration and transparency across diverse stakeholders, including a variety of organizations all along the generative AI supply chain.
Generative AI has made the need for AI governance—the coordination of people, processes, and tools to ensure AI risks are effectively mitigated at every stage of the AI lifecycle and at every layer of the AI stack—clearer than ever.
Enterprises that want to unlock the value of generative AI need to establish processes to evaluate the risks of the AI applications they’re building and buying, adopt controls that mitigate against those risks, and assign accountability for ongoing risk management of AI.
The Governance Layer

At Credo AI, we build tools to help organizations operationalize AI governance.
Our AI Governance Platform is the enterprise command and control center for AI governance at scale, designed to help you ensure that risk-mitigating controls are implemented across your technology stack to support the safe and responsible development and use of genAI tools and applications.
We provide specific guardrails across the entirety of the genAI stack, designed to integrate into the many different tools and applications your organization might be leveraging for GenAI governance. At the infrastructure layer, we provide specific guidance in the form of Policy Packs to guide your implementation of a secure deployment. At the model and application layers, we are tracking the rapidly expanding Ops space and can help you configure the technical guardrails to ensure your system is aligned with your organization’s values. Finally, at the end-user layer, we are the leader in identifying and configuring the processes and technical controls to ensure users at your organization are complying with the policies you set regarding genAI tools.
Whether you’re a foundation model provider, an application developer, or an enterprise trying to adopt genAI tools for end use, effectively managing the risks of generative AI through AI governance is essential to realizing its full potential.
In Conclusion
Governance of generative AI systems is essential to realize their full potential and mitigate their many risks. And as you’ve seen, governance of these systems requires orchestration across different layers of the technology stack across a variety of different tools and applications.
Adopting a strong AI governance program will enable you to manage the complexity of generative AI governance, and leveraging AI governance tools like Credo AI can help you scale these efforts across your entire organization.
Learn more about how Credo AI can help you implement generative AI governance today.
Key Takeaways:
- Many companies are worried about the risks associated with generative AI tools such as ChatGPT and GitHub CoPilot. They feel that without proper governance, they are unable to monitor and control how their employees utilize these tools, which poses risks to their business.
- Organizations that fail to incorporate generative AI tools into their processes and operations may be left behind, while those who effectively harness this technology can gain a competitive advantage and succeed in their markets.
- To ensure the safe and responsible use of generative AI, governance must be implemented at various layers of the generative AI stack. These layers include infrastructure governance, model governance, application layer governance, and end-user governance. Each layer presents opportunities to enforce controls and mitigate risks associated with generative AI systems.
- At Credo AI, we build tools to help organizations operationalize AI governance. Our Responsible AI Governance Platform is the enterprise control center for AI governance at scale, designed to help you ensure that risk-mitigating controls are implemented across your technology stack to support the safe and responsible development and use of genAI tools and applications.
DISCLAIMER. The information we provide here is for informational purposes only and is not intended in any way to represent legal advice or a legal opinion that you can rely on. It is your sole responsibility to consult an attorney to resolve any legal issues related to this information.