Part #2: Operationalizing Responsible AI: How do you “do” AI Governance?
Now that we’ve established what AI governance is and why it’s so important, let’s talk strategy; how does one do AI governance, and what does an effective AI governance program look like? In case you haven't read Part #1: What is AI Governance?, click here.
At the highest level, AI governance can be broken down into four components—four distinct steps that make up both a linear and iterative process:
- Alignment: identifying and articulating the goals of the AI system
- Assessment: evaluating the AI system against the aligned goals
- Translation: turning the outputs of assessment into meaningful insights
- Mitigation: taking action to prevent failure
Let’s take a deeper look at what happens during each of these steps, and how they come together to form a governance process designed to prevent catastrophic failure.
1) Alignment: Translating principles into practice
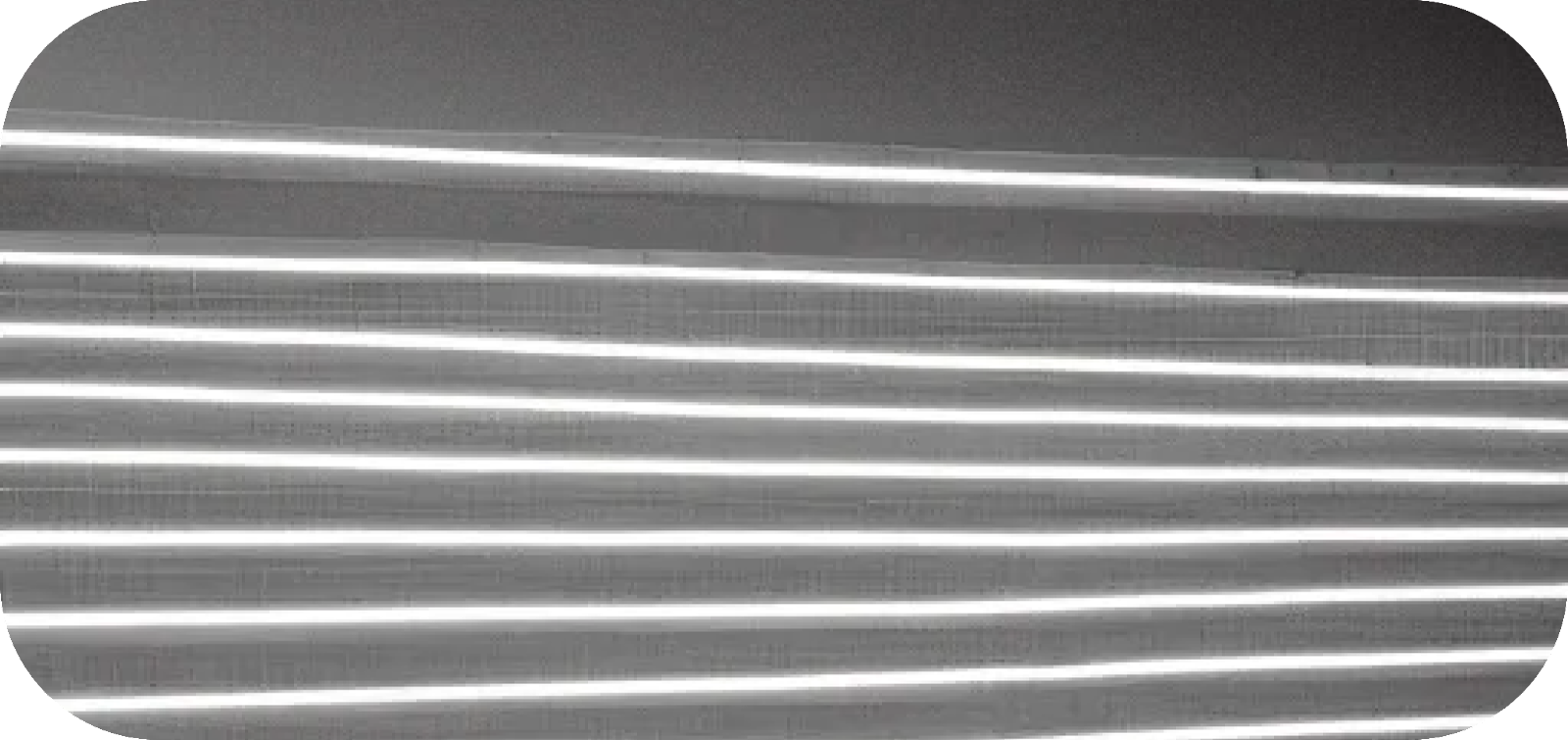
Put simply, the Alignment step of AI governance is about defining requirements for an AI system. An effective set of requirements should include both technical requirements, such as minimum thresholds of performance or specific requirements around parity of outcomes across different groups, and process requirements, such as specific documentation that the development team must produce during each step of the AI lifecycle.
We call this step “alignment” for two reasons:
1) This step defines requirements for the AI system that ensure it is aligned with the principles, values, and goals that we need the system to uphold.
2)I It requires alignment across technical and non-technical stakeholders, who each hold a piece of the puzzle that is necessary to align on the right requirements.
Alignment is one of the most important steps of AI governance, and it is also one of the hardest. There are a few reasons why most organizations and teams find alignment so challenging:
1) This is highly context-dependent and requires diverse sets of skills and expertise.
To make these decisions, you need domain expertise—and not just technical expertise, but also legal, ethical, and cultural understanding to ensure the requirements you define for your system are truly aligned with the needs of your use case. There is no “one size fits all” approach to selecting the right technical or process requirements for an AI system to ensure that it is fair, robust, transparent, etc.
Let’s look at aligning on fairness metrics as an example:
For a hiring algorithm, you may define “fairness” as parity of outcomes of the model, since you want different groups to be selected for employment at similar rates (women vs. men). For a cancer detection system, however, parity of outcomes is meaningless—you don’t care if women are detected as having breast cancer at the same or similar rates as men, but instead, you want to make sure that your system is as accurate as possible for all different groups. So your definition of fairness for this model would be parity of performance across different groups.
While these two scenarios are relatively straightforward to reason through, there are many scenarios where it’s not as clear how one should define “fairness,” “robustness,” or “transparency”—some of the key dimensions of Responsible AI referenced in many regulations and frameworks. Technical stakeholders who understand what can be measured must work together with non-technical stakeholders who understand what is most important for the system to do given the specific business, ethical, and regulatory context in which it’s operating.
2) There aren’t clear standards or benchmarks for most use cases, making it difficult to define “what good looks like.”
Selecting the minimum threshold for a fairness metric, a minimum threshold for an adversarial robustness measure, or the level of detail required for an acceptable explanation of model outcomes isn’t straightforward, even when you have deep domain expertise. While many organizations are contributing to the push for AI standards, we are a long way away from having interoperable standards and guidelines like other more mature technology governance domains, such as cybersecurity or even data privacy.
Arriving at standards and benchmarks requires transparency as a first step. Aligning on what to measure and then transparently reporting on outcomes across different AI applications and industries will help us move towards more mature governance frameworks that include more specific definitions of acceptable bounds.
2) Assessment: Evaluating the system against requirements
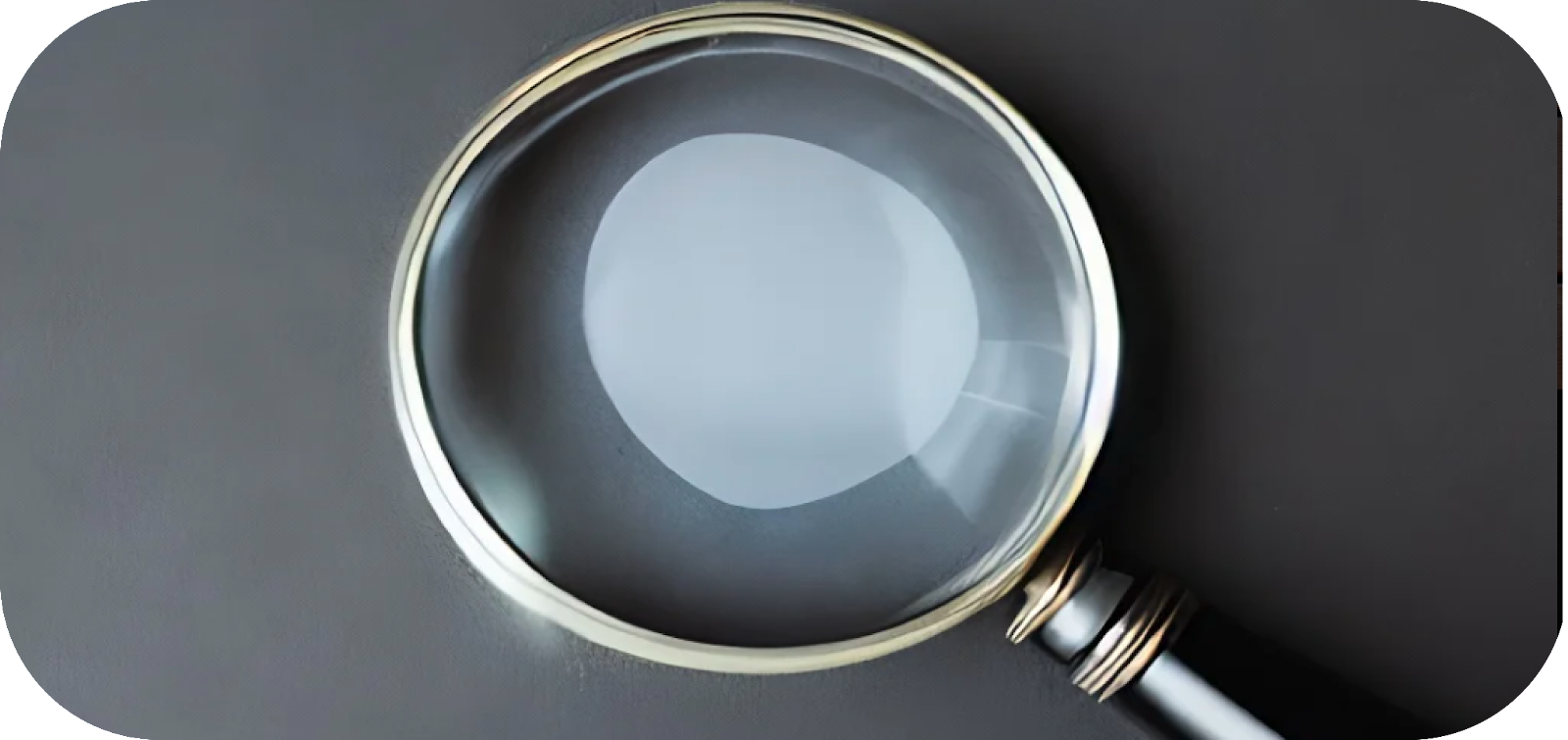
After establishing principles, we need to assess systems against the requirements that were aligned upon. Hence, it is crucial that AI/ML development teams are empowered to measure what needs to be measured about ML models and datasets and to document the processes that need to be implemented for the AI system to be aligned with principles.
There has been a tremendous focus in the last few years on technical tools for technical stakeholders to be able to better evaluate their AI systems for bias, drift, and explainability. These tools are essential for effective assessment—MLOps tools and open-source libraries that offer experiment management, model comparison, and production monitoring are some examples of the tools available to data scientists and ML engineers to understand the behavior of their models throughout the development lifecycle.
Technical assessment, however, is just one part of the comprehensive assessment for AI governance. Remember, our requirements from Alignment should include both technical and non-technical requirements that we need to evaluate to ensure that our system is meeting our needs and expectations. Assessment should include activities like reviewing the harms/benefits analysis that the development team conducted at the start of system design; ensuring that Model Cards and Datasheets are available for end users of the system, and conducting usability analysis of model output explanations with people impacted by the system to ensure that they can understand how it works.
3) Translation: Turning evidence into insights
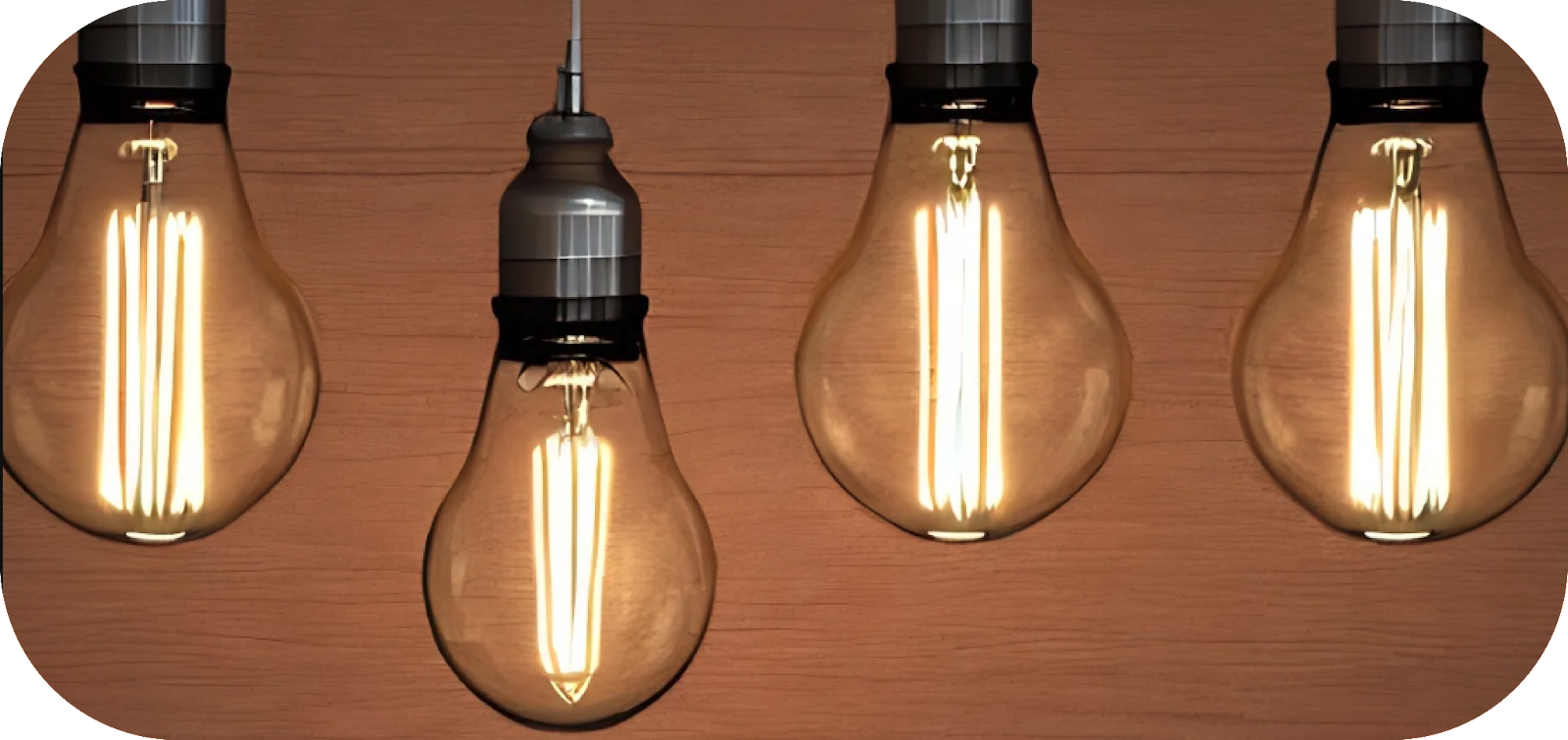
The output of the Assessment phase of governance is a collection of evidence, which will help stakeholders understand where the system is meeting expectations and where it is falling short. Making this evidence meaningful to AI system stakeholders, so they can make decisions about the system, and its responsible use is a critical step in the governance process.
Many of the stakeholders of AI systems are non-technical; the business owners, legal and compliance reviewers, and end users of the system all have a significant stake in understanding whether the system is meeting expectations. These stakeholders, however, may struggle to understand exactly what it means for the system to have a precision of 0.96, or a demographic parity ratio of 0.82. The raw outputs of assessment may not provide the insight needed for these stakeholders to make effective decisions about whether a system is safe to put into production or whether they want to interact with the system in a specific scenario.
Translation of evidence into governance artifacts requires, again, that technical and legal, business, and ethics experts bring together their expertise—a common theme throughout the governance process.
Governance artifacts can take many different forms, depending on the context and need. An internal governance team may favor a dashboard that shows which requirements are passing and which are failing at a quick glance; a regulator or end user may want a transparency report that describes how a system works in plain language, simple for a non-data-scientist to understand; and a legal team may need an audit report that is certified by an independent auditor to meet certain legal or regulatory requirements.
Again, there is no “one size fits all” governance artifact—and most systems will need multiple governance artifacts to effectively translate governance evidence into something meaningful for decision-makers. But this is a step that cannot be forgotten, as it is critical to ensuring that there is a shared understanding of the current state of a system, which is essential for the last and final step of governance.
4) Mitigation: Taking action to prevent failure
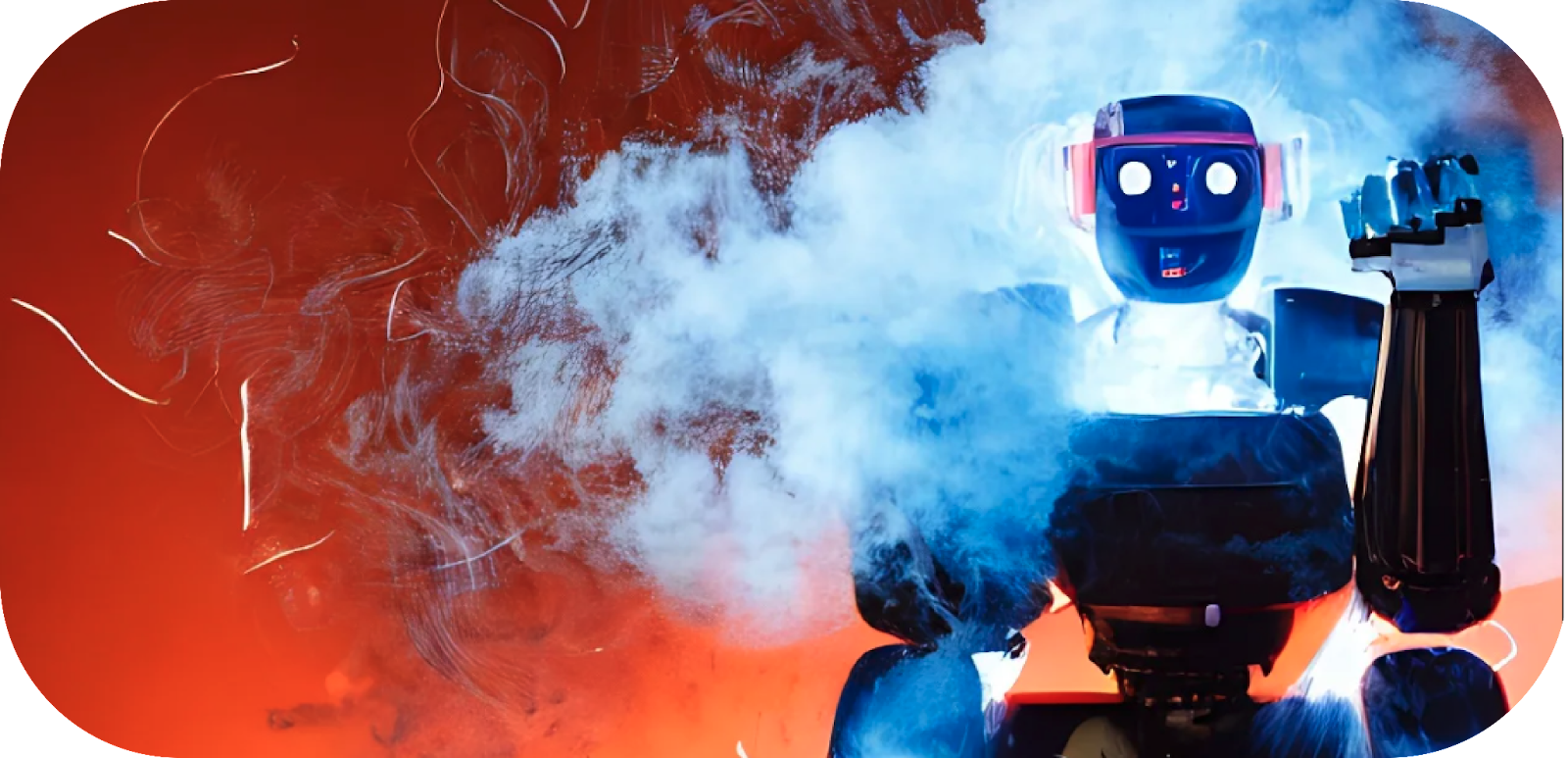
Everything we’ve described about the governance process up until this point has been about gap analysis of an AI system—that is, understanding the current state of a system and where/how it’s meeting or not meeting requirements based on our values and goals. But, as discussed in our introduction, the real purpose of governance is to coordinate actions across a diverse set of stakeholders to prevent catastrophic failure. And so, the final step of the AI governance process is to make decisions based on the current state of a system that are designed to mitigate risk and prevent the system from causing harm.
Just as we saw in Alignment and Assessment, Mitigation spans both technical and non-technical actions.
1) Technical mitigation might look like retraining a model with a more balanced training dataset to reduce unintended harmful bias, or retraining the model with adversarial examples to improve its robustness against attack.
2) Non-technical mitigation techniques might include adding ways for end users to provide feedback and report harmful system errors, or providing paths of recourse for impacted individuals.
And again—as we have seen with all of the previous steps of governance—mitigation is a team sport that spans a set of diverse stakeholders. The risks and challenges of AI systems cannot be solved by any one stakeholder alone but need to be addressed by a community of practitioners who each bring a unique perspective to the table. An effective AI governance program is laser-focused on empowering these practitioners with the insights they need to proactively mitigate risk, before a critical issue occurs.
In Conclusion
We feel like a broken record about now, but hopefully, a few key themes are clear by this point in the post:
1. AI governance takes a village.
Each step of the governance process requires input based on technical expertise, legal expertise, domain knowledge, and ethical understanding. If you are lucky enough to find one person who has all of these skills, congratulations—you’ve found an AI governance unicorn! The much more likely scenario is that you’ll have to bring together a group of people who each have a piece of the puzzle, and they will need to effectively collaborate to execute AI governance. Bringing these stakeholders together and helping them speak each other’s language is the most important thing that you can do to set yourself up for AI governance success.
2. Proactively preventing failure is the goal.
The greatest risk that AI systems pose to humanity is their ability to operate at an almost unimaginable scale—which means that if something goes wrong, it can go very wrong very quickly in ways that may not be fixable. Preventing catastrophic AI failure is imperative not just for the well-being of organizations that are trying to adopt AI, but for societal and human well-being. AI governance is about getting the right insights to decision-makers who can take proactive steps to mitigate AI risk and prevent failure modes.
3. Transparency is the first step.
While AI adoption and governance maturity are still relatively low, defining hard requirements and rules for AI systems is incredibly difficult. The best thing that organizations can do right now to help establish standards is to provide transparency into what they’ve decided to measure and why and the results of those measurements. Over time, the Responsible AI ecosystem will converge on the best practices and approaches that come out of this period of transparency—and we will, eventually, end up with a mature AI governance framework or frameworks akin to what we’ve seen happen in the cybersecurity space.
There are many different roles to be played in helping to create and solidify a culture of governance and responsibility in the AI ecosystem today—and the four steps we’ve outlined above are relevant to a wide variety of different participants.
Whether you’re a standard-setting body creating frameworks and guidelines for AI builders and users; or an organization building and buying AI systems; or a regulator making laws that dictate how AI systems can be built and used, the steps of Alignment, Assessment, Translation, and Mitigation can guide your work and help you establish oversight and accountability.
We are excited to see the AI Governance ecosystem continue to grow and mature, and we are here to support our customers along every step of the AI Governance journey. If you’re interested in learning more about how Credo AI can help your organization build AI governance capacity, please reach out to learn more about our Responsible AI Governance Platform.
🙋Interested in learning how to implement AI governance? Reach out to us at demo@credo.ai.
DISCLAIMER. The information we provide here is for informational purposes only and is not intended in any way to represent legal advice or a legal opinion that you can rely on. It is your sole responsibility to consult an attorney to resolve any legal issues related to this information.